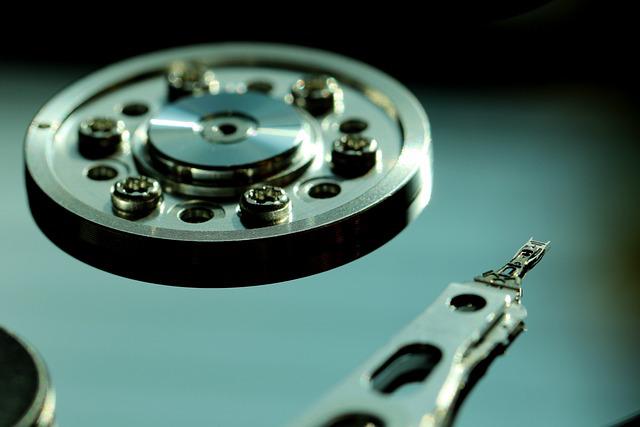
At Instagram, we had many different job roles that analyzed data. A few of the data job titles included: data scientist, analyst, researcher and growth marketing.
There’s often a lot of confusion between the roles of data scientist vs. decision scientist.
We had both at Instagram and they fulfilled different needs, so I thought I’d explain the main differences I see from my personal experience in the decision science role, working closely with my data science colleagues.
Data Science vs. Decision Science
The data scientist focuses on finding insights and relationships via statistics. The decision scientist is looking to find insights as they relate to the decision at-hand. Example decisions might include: age groups on which to focus, the most optimal way to spend a yearly budget or how to measure a non-traditional media mix. For decision scientists, the business problem comes first; analysis follows and is dependent on the question or business decision that needs to be made.
Data Scientists vs. Decision Scientists: How to Think About Data
Thinking drives action, so I’ll compare each role by reviewing the way data scientists and decision scientists differ in terms of thinking about data.
Data Scientists
Data is the Tool for Improving and Developing New Products Based on Robust Statistical Methods
Data scientists are looking to understand, interpret and analyze with the goal of building better products. Therefore, data quality, statistical rigor and measurement perfection are often their trademarks.
For data scientists, the analysis, statistical rigor and understanding comes first. Business challenges come second.
Data scientists think about data in terms of data patterns, data processing, algorithms and statistics. Often, data scientists are conducting deep analysis and experimental statistics. They are obsessed with finding causal relationships.
Data scientists are deeply focused on data quality as it relates to their product area because better data quality results in more thorough statistical analysis.
Data scientists frame data analysis in terms of algorithms, machine learning, statistics and experimentation. They are looking to bring order to big data to find insights and learnings as they relate to their product or focus area. They have a statistics …….